DystoniaBoTXNet: Novel AI Model Predicts Botulinum Toxin Efficacy for Individual Patients With Isolated Dystonia
Key findings
- DystoniaBoTXNet, developed at Mass Eye and Ear, is a deep learning algorithm designed to predict whether an individual with isolated dystonia will respond to botulinum toxin (BoTX) based on input from a brain MRI
- DystoniaBoTXNet was trained on 165 patients with laryngeal dystonia and tested on 90 independent patients with laryngeal dystonia, blepharospasm, cervical dystonia, or writer's cramp
- The model was 96% accurate in differentiating between previously documented responders and nonresponders to BoTX; its sensitivity was 100% and its specificity was 86%
- DystoniaBoTXNet was also tested on 29 BoTX-naïve patients with laryngeal dystonia; it found that 23 patients had a 95% probability of responding to BoTX and that six patients had an 83% probability of not responding to treatment
- The deep learning neural network may be effective for selecting candidates for initial BoTX treatment and refined decision-making about additional injection cycles when patients don't respond to the first injection
Botulinum toxin (BoTX) injection into affected muscles is the first-line therapy for isolated dystonia. However, establishing efficacy requires an average of three to four treatment cycles, each lasting several months, to test different injection regimens.
Subscribe to the latest updates from Otolaryngology Advances in Motion
Besides being cost-inefficient, this trial-and-error approach may lead to overtreatment of patients who will never respond and undertreatment of patients who discontinue before they do respond.
Kristina Simonyan, MD, PhD, Dr med, director of Laryngology Research at Mass Eye and Ear, and colleagues Dongren Yao, PhD, and Lena C. O'Flynn have developed a deep learning algorithm, DystoniaBoTXNet, that predicts whether an individual with isolated dystonia will respond to BoTX. They describe the new model in the Annals of Neurology.
Methods
The study included patients with isolated focal dystonia who received BoTX type A injections when they were fully symptomatic (at least three months after their last injection):
- Training set—165 patients with laryngeal dystonia (106 responders to BoTX and 59 nonresponders); laryngeal dystonia is the most common type of dystonia treated with BoTX
- First independent set—44 different patients with laryngeal dystonia (29 responders and 15 nonresponders)
- Second independent set—46 patients with blepharospasm (n=14), cervical dystonia (n=18), or writer's cramp (n=14); 38 were responders and eight were nonresponders
All patients underwent structural brain MRI. Scans from patients in the training set were used to develop and test DystoniaBoTXNet, a convolutional neural network that can "learn" to interpret images. The training model achieved an AUC of 100% in discriminating between responders and nonresponders.
DystoniaBoTXNet identified clusters in superior parietal lobule, inferior and middle frontal gyri, middle orbital gyrus, inferior temporal gyrus, corpus callosum, inferior fronto-occipital fasciculus and anterior thalamic radiation as biomarkers that can predict response or nonresponse to BoTX. These regions are known to contribute to dystonia pathophysiology, and they are implicated in the central effects of BoTX.
Testing
When MRI scans from patients in the independent sets were input into DystoniaBoTXNet:
- First set—95% accuracy in predicting BoTX benefit; 11% of patients referred for further evaluation; AUC, 94%; sensitivity, 100%; specificity, 85%; positive predictive value, 93%; negative predictive value, 100%
- Second set—98% accuracy; 4% referral rate; AUC, 92%; sensitivity, 100%; specificity, 87.5%; PPV, 97%; NPV, 100%
- Overall performance—96% accuracy; sensitivity, 100%; specificity, 86%; referral rate, 8%
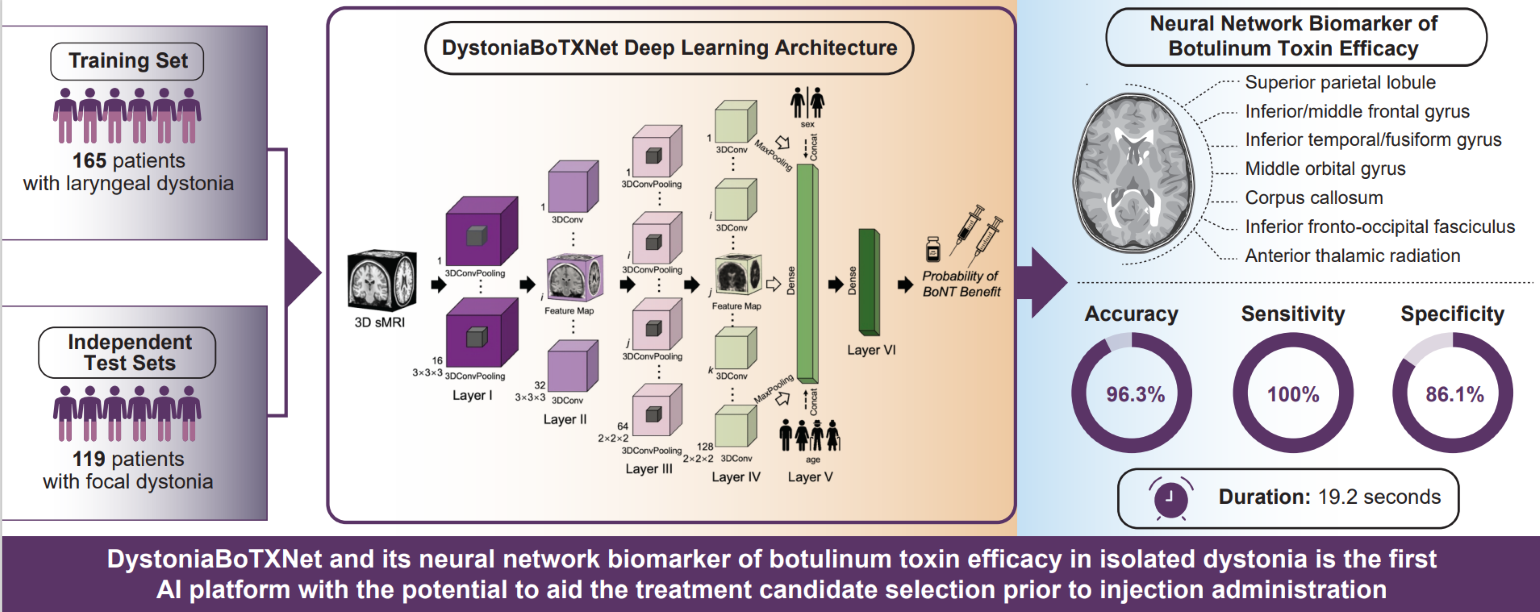
Figure 1
DystoniaBoTXNet and its neural network biomarker of botulinum toxin efficacy in isolated dystonia is the first AI platform with the potential to aid the treatment candidate selection prior to injection administration. Image courtesy of Kristina Simonyan, MD, PhD, Dr med.
Translational Potential
The researchers also studied 29 BoTX-naïve patients with laryngeal dystonia who received BoTX and were followed prospectively:
- 23 patients had a 95% probability of responding to BoTX; six patients had an 83% probability of not responding to treatment
- Five patients (four adductor and one abductor types) who received BoTX after study participation and responded to the treatment—DystoniaBoTXNet was 100% accurate in predicting benefit
- Two patients (abductor type) who received BoTX after study participation and did not respond—DystoniaBoTXNet was false-positive in predicting benefit (91% median probability of benefit)
- Both patients underwent only one injection cycle and declined subsequent treatment; they may have been pseudo-nonresponders
The average computational time of DystoniaBoTXNet was 19 seconds per case.
Interpreting the Findings
Given its speed and accuracy, DystoniaBoTXNet incomparably outperforms the current empirical approach of establishing BoTX benefits. It may be effective for selecting candidates for BoTX treatment initiation and shared decision-making about additional injection cycles when patients don't respond to the first.
view original journal article Subscription may be required
Learn more about the Department of Otolaryngology–Head and Neck Surgery at Mass Eye and Ear
Refer a patient to Mass Eye and Ear