New Artificial Intelligence Platform Diagnoses Dystonia With Near-Perfect Accuracy
In This Article
- Dystonia is a disabling neurological condition that can take up to 10 years to diagnose. Since most dystonia cases have no known cause nor objective biomarker, clinicians are often limited to a subjective assessment of symptoms to diagnose the disorder
- Researchers at Mass Eye and Ear have developed an Artificial Intelligence (AI)-based deep learning platform called DystoniaNet, which is the first technology of its kind to provide an objective diagnosis of the disorder
- The platform compared the brain MRIs of 612 people, including 392 patients with three different forms of isolated focal dystonia and 220 healthy individuals. DystoniaNet diagnosed dystonia with 98.8% accuracy in less than a second per case
- A new microstructural neural network biological marker of dystonia was identified in the process
- Testing of the deep learning platform has already moved into clinical settings at Mass Eye and Ear and Massachusetts General Hospital
Subscribe to the latest updates from Otolaryngology Advances in Motion
Dystonia is a neurological condition that causes involuntary muscle contractions. The majority of cases are isolated, which means they affect a single muscle group, and lead to abnormal movements and postures. In some instances, symptoms are so severe that patients become permanently disabled.
Up to 3.5 out of every 100,000 people have isolated dystonia, a figure experts widely believe to be an underestimate. Often without a clear cause, the condition is extremely hard to diagnose. Its wide variability of symptoms, which can range from whole body twisting to difficulty speaking, and the absence of a universal biomarker, can easily disguise the disorder as another neurological condition.
Not only does dystonia diminish a patient’s quality of life, but the lack of diagnostic measures prevents them from receiving appropriate care and timely treatment.
“Dystonia has frustrated clinicians and researchers for decades,” explained Kristina Simonyan, MD, PhD, Dr med, director of Laryngology Research at Mass Eye and Ear and associate professor of Otolaryngology–Head and Neck Surgery at Harvard Medical School. “Without an objective measure of diagnosing the disorder, physicians often rely on a process of elimination to rule out dozens of similar conditions before arriving at a dystonia diagnosis.”
A deep-learning platform called DystoniaNet is giving clinicians a reason to believe a reliable, diagnostic tool for dystonia could soon be on the horizon. Recently developed by Dr. Simonyan and a postdoctoral fellow in her lab, Davide Valeriani, PhD, the platform can detect dystonia from an MRI scan of the brain with near-perfect accuracy.
By using its training algorithm to identify a microstructural neural network in a patient’s MRI, DystoniaNet can objectively detect the disease, derive an accurate diagnosis and ultimately change the way clinicians treat the disease for years to come.
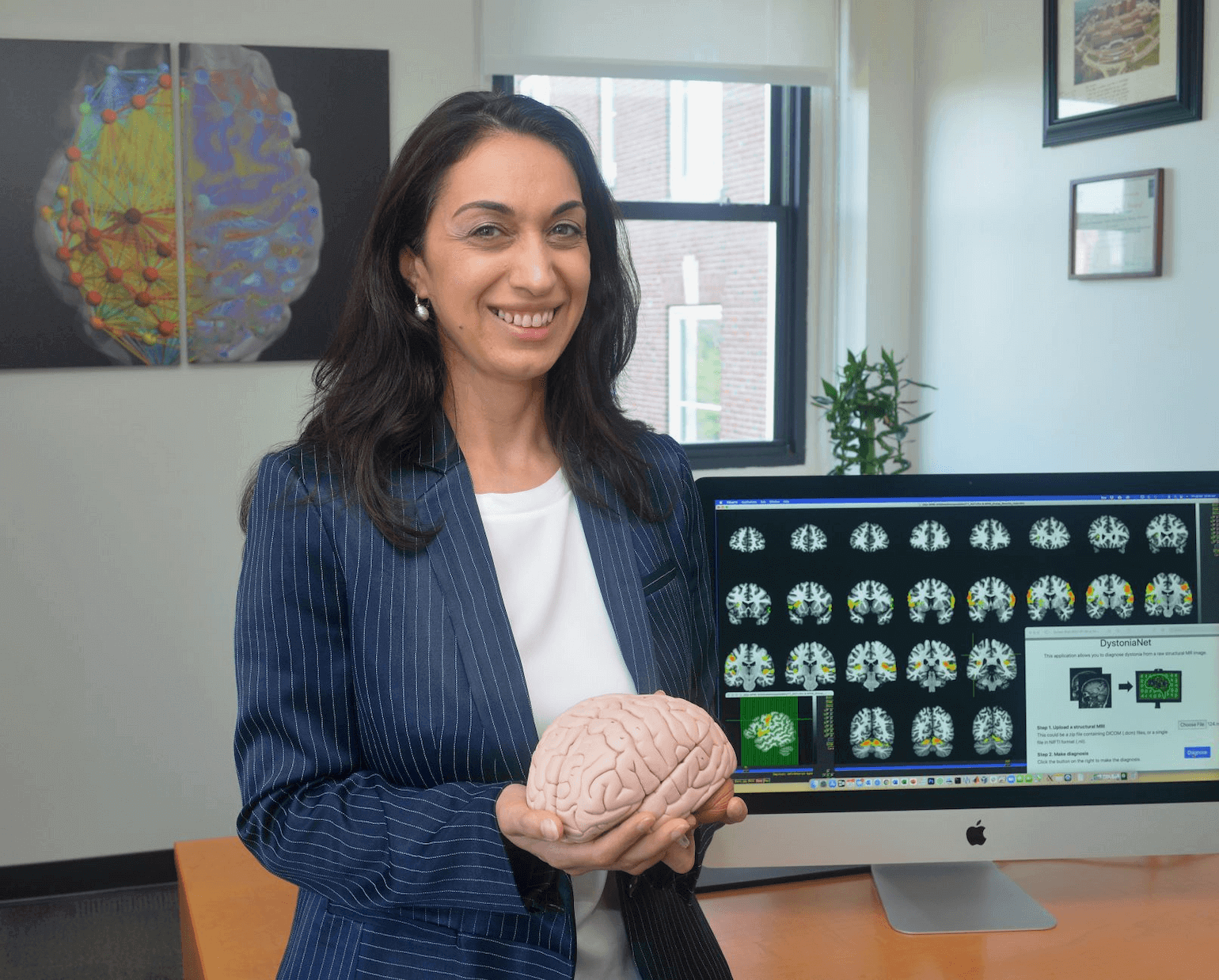
Kristina Simonyan, MD, PhD, Dr med, director of Laryngology Research at Mass Eye and Ear
An Objective, Fast and Accurate Diagnostic Tool
A 2018 study revealed that the agreement rate between clinicians on the diagnosis of laryngeal dystonia is low. Following subjective evaluation of a patient’s symptoms, expert clinicians arrived at the same diagnosis only about 34% of the time. Inconsistent evaluations based on a physician’s expertise and experiences with dystonia often lead to conflicting opinions, and nearly 50% of cases are misdiagnosed.
“Subjectively assessing symptoms is not a sufficient way of determining a dystonia diagnosis,” said Dr. Simonyan. “Since dystonia is a neurological disease, we need to look at the problem from the perspective of underlying brain alterations.”
DystoniaNet does exactly that. The platform uses an algorithm to analyze data collected from individual MRI scans. By identifying subtle structural differences, the algorithm can detect abnormal clusters in several regions of the brain that are known to contribute to the pathophysiology of dystonia. These small changes cannot be seen by the naked eye—they can only be seen through the platform’s ability to zoom into the microstructural details of the MRI.
In a recent study published in Proceedings of the National Academy of Sciences in the United States of America, DystoniaNet helped diagnose dystonia with an average accuracy of 98.8%. The platform analyzed the MRI scans of 612 people enrolled in the study and arrived at a diagnosis in less than one second. Of those enrolled, 392 people were patients with one of the three most common types of isolated dystonia—laryngeal dystonia, cervical dystonia and blepharospasm—and 220 were healthy individuals.
Remarkably, the platform accurately diagnosed 100% of cervical dystonia cases, 98.2% of laryngeal dystonia cases and 98.1% of blepharospasm cases. Only six patients were referred for further examination.
A Biomarker Capable of Streamlining Clinical Care
On average, it can take up to 10 years for a patient to receive a correct dystonia diagnosis. According to Nutan Sharma, MD, PhD, associate professor of Neurology at Harvard Medical School and director of the Dystonia Clinic at Massachusetts General Hospital, a person might visit a primary care physician at the onset of symptoms, only to be referred to a physical therapist. When physical therapy fails, the patient might see several other specialists before a diagnosis is confirmed. Eventually, a movement disorder specialist is needed to manage the irregular muscle contractions with a combination of botulinum toxin injections and oral medication.
DystoniaNet has the potential to streamline this process so that a person can travel straight from a primary care doctor to a movement disorder specialist within days or weeks.
“If you’re living in a rural area hundreds of miles away from a research hospital, a reliable diagnostic test could save a person years of their life trying to understand what is causing their symptoms,” said Dr. Sharma.
Theoretically, a physician would upload a patient’s MRI to the DystoniaNet platform and, with the click of a button, generate a metric that uses AI to determine whether the patient should immediately visit a movement disorder specialist for a follow-up on a dystonia diagnosis. The metric generated by DystoniaNet wouldn’t be the lone variable in determining a diagnosis, however. Clinicians would need to evaluate the metric alongside a slew of other variables such as medical history, symptoms and medications. Dr. Simonyan compared the metric to that of a blood test for the diagnosis of diabetes.
“Blood work alone isn’t enough to determine a diagnosis for diabetes, and DystoniaNet isn’t designed to deliver a final diagnosis for dystonia,” she said. “Rather, it is one powerful variable being weighed alongside the patient’s history and evaluation of symptoms.”
The Next Steps for DystoniaNet
Funded by a R01 grant from the National Institutes of Health (NIH) and in part by philanthropic gifts from Keith and Roberta Richardson and Amazon Web Services, Dr. Simonyan’s research on DystoniaNet has garnered attention around the world.
In January of 2021, Dr. Simonyan was awarded a Mass General Brigham Innovation Discovery Grant to continue her research. Working in collaboration with Dr. Sharma and Phillip C. Song, MD, director of the Division of Laryngology at Mass Eye and Ear, Dr. Simonyan and a team of researchers will test the efficacy of her deep-learning platform in clinical settings at Mass Eye and Ear and Mass General.
Outside the clinic, DystoniaNet has already changed how researchers assess the disorder. According to Laurie Ozelius, PhD, associate professor of Neurology at Harvard Medical School, the platform confirms that dystonia affects communication between different structures of the brain, as opposed to the function of a single brain structure.
“Unlike Huntington’s Disease, where there’s a clear structural abnormality in one part of the brain, dystonia is a circuit disease where the abnormality lies in how different parts of the brain interact,” Dr. Ozelius said. “Once we understand why these signals misfire and how we can get them to work together again, we would move one step closer to a possible long-term treatment.”
As research on dystonia expands, DystoniaNet will continue to play an integral role in diagnosing patients, understanding its underlying causes and pioneering a cure.
Read More on Fall 2021 Harvard Otolaryngology Magazine
Learn more about the Department of Otolaryngology–Head and Neck Surgery at Mass Eye and Ear
Refer a patient to Mass Eye and Ear