Artificial Intelligence Enters the Ear
In This Article
- Mass Eye and Ear clinicians have built an artificial intelligence (AI) model capable of diagnosing ear infections from photos taken on a handheld device
- The clinicians updated an earlier, highly-accurate model with more than 639 surgical images of eardrums from children aged 18 years or younger
- The added label differentiated between infected fluid and non-infected fluid. Even with the more complex analysis, the model achieved a mean diagnostic accuracy of 80.8%
- The machine learning model correctly categorized more than 95% of the sample images, whereas the average diagnostic score among 39 clinicians was 65%
Subscribe to the latest updates from Otolaryngology Advances in Motion
Few public health issues affect children more than middle ear infections. In the United States alone, many parents cite ear infections as a leading reason for a visit to the pediatrician. Ear infections extend far beyond national borders, too. In fact, approximately three of every four children worldwide will contract an ear infection before the age of three.1,2,3
Most infections produce mild symptoms such as ear pain, hearing loss, and a low-grade fever, yet are easily treated with antibiotics. Inadequate timing and accessibility of treatment, however, carry serious consequences. Untreated children can develop chronic hearing loss that may result in delayed language development. In underdeveloped nations where antibiotics are scarce, the most severe infections can result in meningitis, as well as tens of thousands of deaths per year.4 Conversely, antibiotic resistance can occur among children overtreated with antibiotics, rendering medications ineffective against future infections.
An inaccurate diagnosis further complicates treatment. Despite technological innovations and clinical practice guidelines, studies suggest the conventional diagnostic accuracy of ear infections in children from a physical exam remains below 70%.
"During these evaluations, you're asking a cranky, sick child to hold still while trying to look through a handheld device with a window lens less than three millimeters in diameter," explained Matthew G. Crowson, MD, an otolaryngologist at Mass Eye and Ear and assistant professor of Otolaryngology–Head and Neck Surgery at Harvard Medical School. "Even if you're lucky enough to have a child cooperate, the small view and—in some cases—the clinicians' relative inexperience in diagnosing middle ear disease are enough for an incorrect diagnosis."
A heavy burden ultimately falls on parents who, without any easy way of looking inside the ear, must decide whether to seek urgent care for their child in the first place or wait and see if symptoms abate. At Mass Eye and Ear, Dr. Crowson has collaborated with surgeons Michael S. Cohen, MD, assistant professor of Otolaryngology–Head and Neck Surgery at Harvard Medical School, and Christopher J. Hartnick, MD, MS, professor of Otolaryngology–Head and Neck Surgery at Harvard Medical School, to build an artificial intelligence (AI) model capable of diagnosing ear infections from photos taken on a handheld device.
The team's most recent success has been very promising. In a 2022 study published in Otolaryngology–Head and Neck Surgery, their AI model outperformed doctors in diagnosing ear infections, offering a glimpse of what peace of mind might look like for countless parents and clinicians alike.
Looking Past the Eardrum
The majority of ear infections occur in the middle ear, where the smallest bones in the body relay sound from the eardrum to the inner ear. The bones form a delicate chain confined to an empty space: an optimal environment for bacteria to fester.
Children are more susceptible to ear infections than adults. When air pressure spikes inside the middle ear, a special valve opens a narrow tube to the nose. The tube—known as the Eustachian tube—helps equalize pressure between the middle ear and external environment. It can also inadvertently serve as a passageway for bacteria-laden fluid to flow into the middle ear. In children, the Eustachian tubes are shorter and at more of a horizontal angle than those found in adults, which allows fluid to pool into the ear with great ease. Weaker muscles surrounding the tubes can also prevent the valves from opening wide enough to drain the accumulated liquid.
When evaluating the inside of the ear for an infection, some front-line clinicians do not have the tools or experience needed to definitively determine if fluid exists behind the eardrum. In most settings, these clinicians use a handheld otoscope to view the outside of the eardrum. A definitive diagnosis, however, requires a less routine practice: testing the infected fluid behind the eardrum by extracting it from an incision.
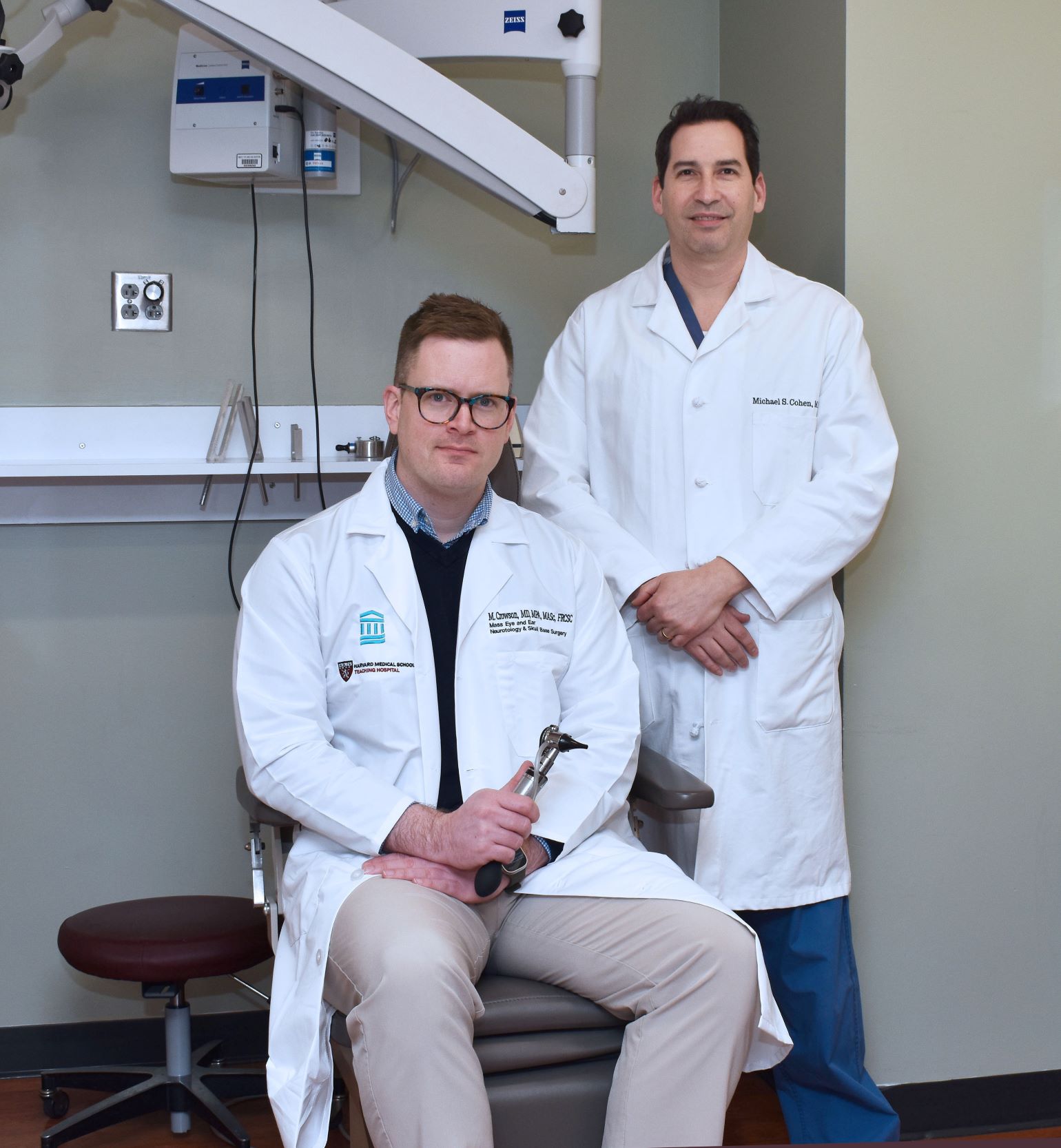
Figure 1
Michael S. Cohen, MD, stands beside Matthew G. Crowson, MD. Image courtesy of Garyfallia Pagonis.
At Mass Eye and Ear, Drs. Cohen and Hartnick have the opportunity to extract such samples during ordinary ear tube insertions for children experiencing frequent ear infections. In 2018, the surgeons sought to make the most of the routine procedure. By taking high-resolution, up-close photos of the eardrum before and after the incision and testing fluid extracted from the inner ear, the surgeons figured they could build a unique dataset that would match the external appearance of an eardrum with its respective fluid samples. A large enough collection of photos labeled "infected" or "healthy," could then train an AI model to detect infections from images captured by a regular otoscope.
"AI tools are only as good as the data you feed them, and most tools have only been trained with images pulled from online search engines," said Dr. Cohen, who serves as director of the Multidisciplinary Pediatric Hearing Loss Clinic at Mass Eye and Ear. "We wanted to go straight to the source and gather the gold standard, 'ground truth' data that few other models—if any—had ever accessed."
Unprecedented Accuracy
Over the span of a few years, Drs. Cohen and Hartnick had gathered hundreds of photos from children undergoing surgery for tube insertions. Unfortunately, neither surgeon knew how to build the machine-learning algorithm needed to put the accumulated data to good use. Few surgeons did, which prompted Drs. Cohen and Hartnick to explore possible collaborations with AI specialists outside Mass Eye and Ear. The trajectory of their work changed when Dr. Crowson arrived at Mass Eye and Ear in 2020. His research and expertise in data analytics and AI caught their attention within days of his arrival.
"AI is in its infancy within otolaryngology, let alone the entire medical community, and here was an ear, nose, and throat surgeon who knew how to build elaborate AI models," said Dr. Hartnick, who also serves as director of the Division of Pediatric Otolaryngology at Mass Eye and Ear. "It was as if we had hit the lottery. Everything we needed to create a diagnostic model was here under one roof."
The collaboration between Dr. Crowson and Drs. Cohen and Hartnick paid immediate dividends. Within months, Dr. Crowson would train an artificial neural network to diagnose infections using the surgeons' photographs. In a 2021 proof-of-concept study published in Pediatrics, the model was 84% accurate in detecting "normal" versus "abnormal" middle ears.
Before comparing the accuracy of their model against clinicians, the team would need to refine its dataset. According to their most recent paper, the surgeons updated the model with more than 639 surgical images of eardrums from children aged 18 years or younger. The images were tagged as either "normal," "infected," or having "fluid," as opposed to the "normal" or "abnormal" classifications used in their earlier model. The added label differentiated between infected fluid, which requires antibiotics, and non-infected fluid, which does not. Even with the more complex analysis, the model achieved a mean diagnostic accuracy of 80.8%.
A survey was then created asking clinicians and trainees of various medical specialties to view 22 images of eardrums and to diagnose the ear as one of the three tagged categories. While the machine learning model correctly categorized more than 95% of the sample images, the average diagnostic score among 39 clinicians who responded to the survey was 65%.
Forging a New Reality for Public Health
In collaboration with Mass General Brigham Innovation, Drs. Cohen and Hartnick are currently engineering a handheld tool* that would use the algorithm to help clinicians diagnose ear infections in seconds. The machine learning algorithm is currently employed in a prototype device paired with a smartphone app. Acting as a "mini otoscope," the device would fit over the phone's camera and allow clinicians and parents to take photos of the inside of a child's ear, upload them directly to the app, and receive an estimated diagnosis in seconds.
A final product could have major implications on global health, Dr. Cohen insisted.
"One interesting thing about international medicine is that you can go to a country with no medical infrastructure, but everyone there has a cell phone," he said. "Developing diagnostic tools into smartphone apps could extend medical care to people living in some of the most medically inaccessible parts of the world."
Advances in synthetic data could help refine the prototype even further.
In 2023, Dr. Crowson worked with Krish Suresh, MD, a resident in the Harvard Combined Residency Program in Otolaryngology–Head and Neck Surgery, to develop a separate machine learning model capable of producing new images of eardrums augmented from preexisting, real images. Findings published in PLOS Digital Health revealed that the augmented images were nearly indiscernible from real ones. A study in JAMA Otolaryngology–Head & Neck Surgery went another step further, revealing that augmented images could actually help increase the accuracy of a diagnostic model.
"AI will never replace the time-tested expertise of trained clinicians," Dr. Crowson said. "Instead, if we can learn how to validate and harness these tools, we can transform the way we treat tomorrow's patients and train new clinicians."
*This tool is based on technology Drs. Cohen and Hartnick have developed at Mass Eye and Ear. The technology is currently being licensed by Mass Eye and Ear to a company that Drs. Cohen and Hartnick are forming and in which they have a financial interest.
Citations:
1 Teele DW, Klein JO, Rosner B. Epidemiology of Otitis Media During the First Seven Years of Life in Children in Greater Boston: a Prospective, Cohort Study. J Infect Dis. 1989;160:83–94. doi: 10.1093/infdis/160.1.83.
2 Gunasekera, H., Haysom, L., Morris, P. and Craig, J., 2008. The Global Burden of Childhood Otitis Media and Hearing Impairment: a Systematic Review. Pediatrics, 121(Supplement_2), pp.S 107-S107.
3 Danishyar A, Ashurst JV. Acute Otitis Media. [Updated 2022 Dec 11]. In: StatPearls [Internet]. Treasure Island (FL): StatPearls Publishing; 2023 Jan.
4 Monasta, L., Ronfani, L., Marchetti, F., Montico, M., Vecchi Brumatti, L., Bavcar, A., Grasso, D., Barbiero, C. and Tamburlini, G., 2012. Burden of Disease Caused by Otitis Media: Systematic Review and Global Estimates. PloS One, 7(4), p.e36226.
Learn more about the Department of Otolaryngology–Head and Neck Surgery at Mass Eye and Ear
Refer a patient to Mass Eye and Ear/Mass General Brigham