Artificial Intelligence Improves Diagnosis of Pediatric Middle Ear Effusion
Key findings
- Misdiagnosis of pediatric otitis media has been a persistent problem for decades, and both undertreatment and overtreatment can have serious consequences
- Using intraoperative data, researchers at Massachusetts Eye and Ear trained a neural network to classify tympanic membrane photographs of pediatric patients as "normal" (no effusion) or "abnormal" (effusion present)
- The average image classification accuracy in determining an abnormal or normal ear was 84%, the area under the receiver operating characteristic curve was 0.93 and the F1 score was 0.80
- The research team is now working to train an algorithm with a mid- or low-resolution camera, similar to those found in consumer-grade smartphones and tablets, for use in office visits and at home
Misdiagnosis of pediatric otitis media has been a persistent problem for decades. According to several studies, accuracy doesn't surpass 70% consistently even when otolaryngology care is factored in.
Subscribe to the latest updates from Otolaryngology Advances in Motion
Several research groups have developed convolutional neural networks, a form of artificial intelligence, to aid in establishing the diagnosis. Such computer algorithms learn patterns in visual data labeled by human experts, then are used to evaluate previously unseen data.
Most of these algorithms have been trained to classify pathology using otoscopic images taken without confirmation that an effusion was present. Matthew G. Crowson, MD, MPA, MASc, an otolaryngologist at Mass Eye and Ear, Michael S. Cohen, MD, a pediatric otolaryngologist at Mass Eye and Ear, Christopher J. Hartnick, MD, MS, also a pediatric otolaryngologist at Mass Eye and Ear, and colleagues instead used "ground-truth" data, developing an algorithm based exclusively on intraoperative photographs.
In Pediatrics, the team reports a high rate of accuracy for their model and their plans to adapt it for use by parents and primary care clinicians.
Methods
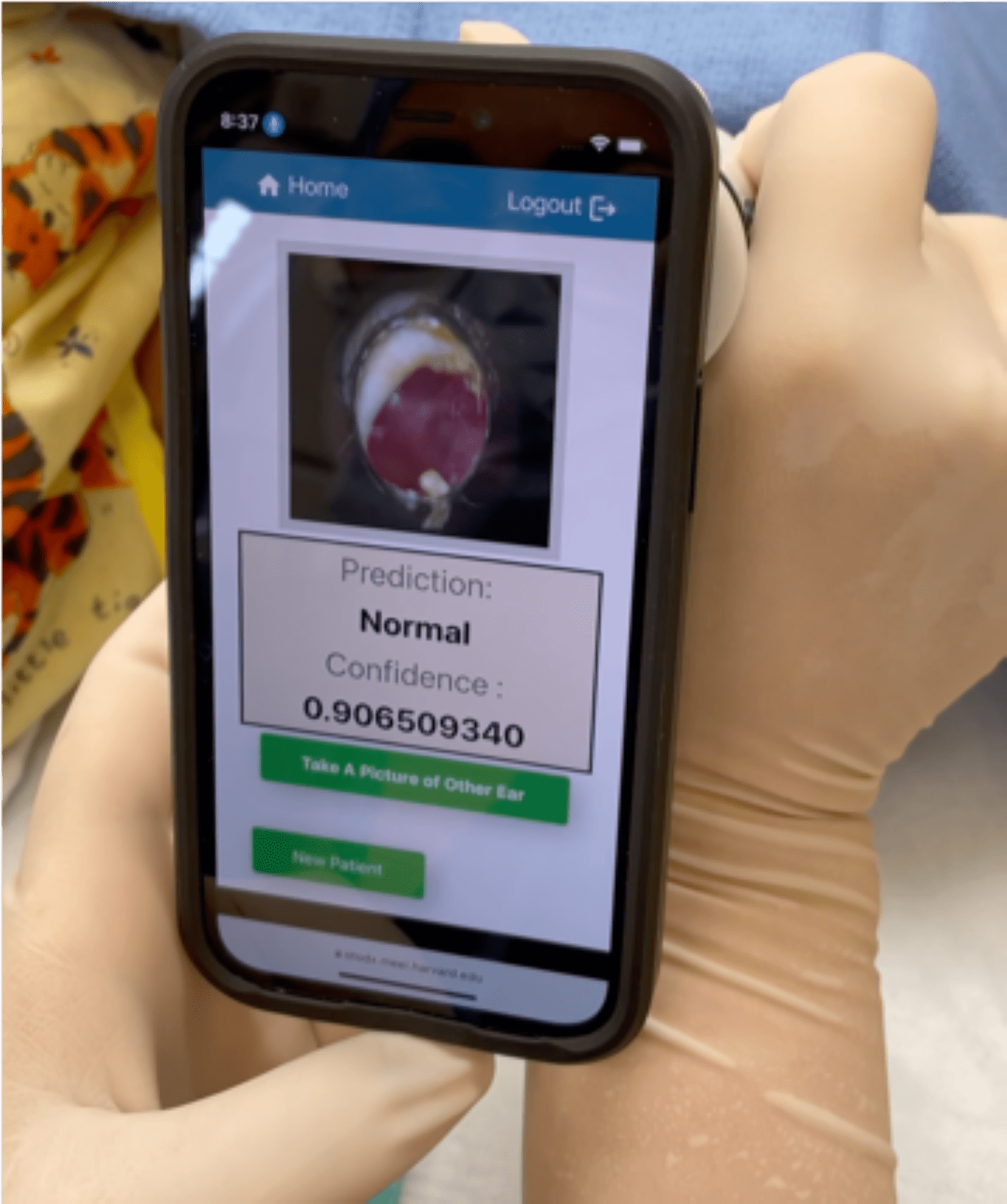
Figure 1
The OtoDX machine learning tool in use, confirming a normal middle ear prior to surgery. Image courtesy of Matthew G. Crowson, MD, MPA, MASc, Michael S. Cohen, MD, and Christopher J. Hartnick, MD, MS.
The researchers took endoscopic high-resolution photographs of the tympanic membranes of children who were being examined under anesthesia for myringotomy and possible tube placement.
By applying a neural network learning framework known as ResNet-34, the team was able to use a relatively small data set, just 267 images, to train their algorithm. Its task was to classify images as "normal" (no effusion) or "abnormal" (effusion present). The algorithm was tested on 68 other intraoperative ear photos that were held out of the training set.
Results
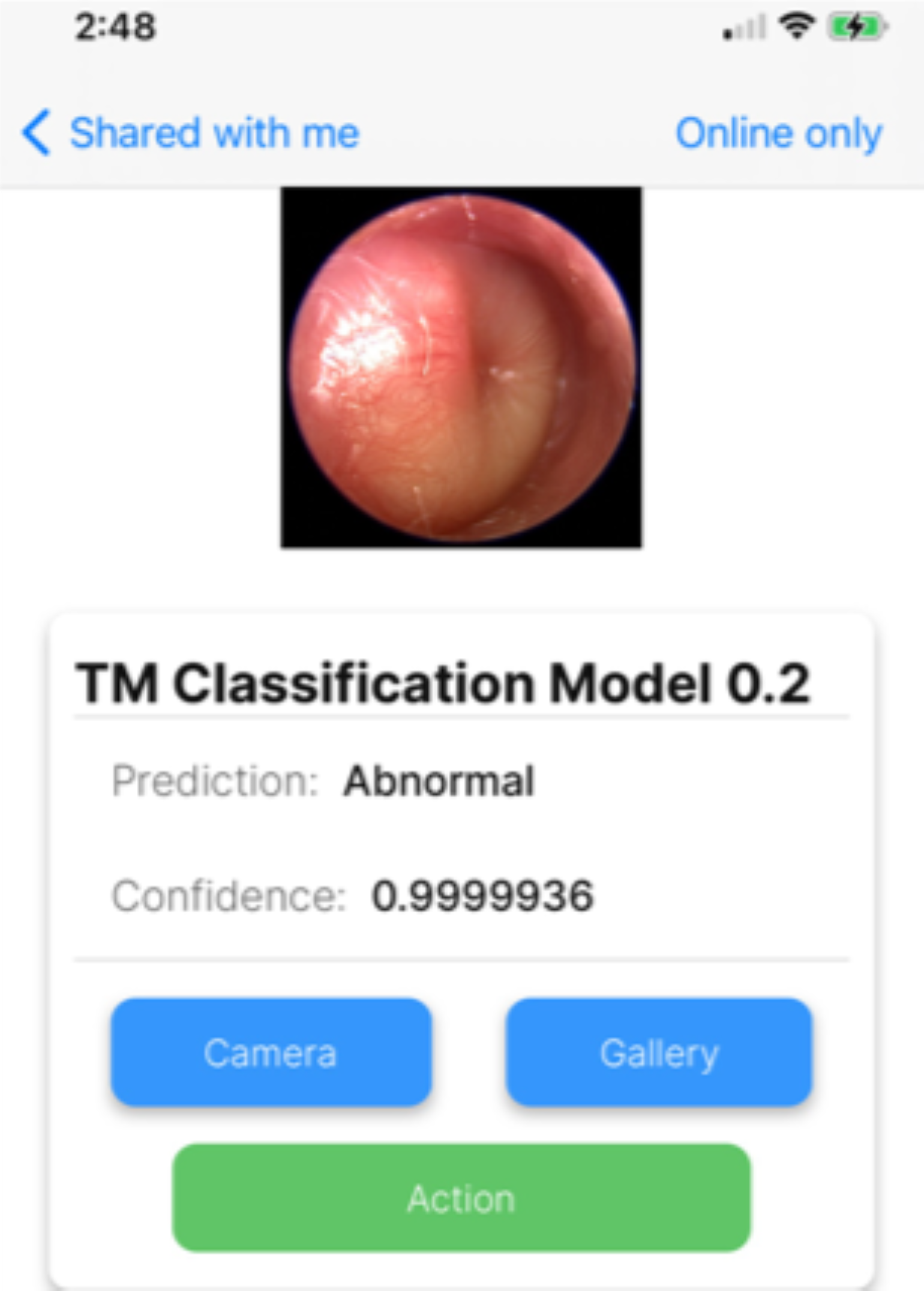
Figure 2
The OtoDX correctly identifies an ear infection. Image courtesy of Matthew G. Crowson, MD, MPA, MASc, Michael S. Cohen, MD, and Christopher J. Hartnick, MD, MS.
The model was highly useful in predicting the presence of an abnormal ear:
- Average image classification accuracy—84%
- Area under the receiver operating characteristic curve—0.93
- F1 score—0.80
The F1 score is the weighted average of a classification model's positive predictive value and sensitivity, with 1 indicating perfect values of both.
Next Steps
In its current state, the neural network isn't ready for deployment. The training data was captured in a highly controlled setting with high-tech equipment, and what's needed is a diagnostic tool that's easy to use during office visits and in patients' homes.
The researchers are working to train an algorithm with a mid- or low-resolution camera, similar to those found in consumer-grade smartphones and tablets. They will also develop training materials for parents about how to accurately take pictures of a child's tympanic membranes.
view original journal article Subscription may be required
Learn more about the Department of Otolaryngology–Head and Neck Surgery at Mass Eye and Ear
Refer a patient to Mass Eye and Ear/Mass General Brigham