"Brain Mapping" to Guide Depression Treatment Not Recommended for Routine Clinical Use at Present
Key findings
- Quantitative electroencephalography (QEEG) is being commercially promoted as a way to predict treatment response in patients with major depression
- In a meta-analysis of all biomarkers considered together, QEEG had reasonable predictive power
- However, no specific biomarker or treatment type showed significantly greater predictive power than any other
- Most studies analyzed did not meet prespecified quality metrics
- The apparent effectiveness of QEEG seems to be an artifact of study design and selective publication
"Brain mapping" with quantitative electroencephalography (QEEG) is being commercially promoted as a way to predict response or nonresponse to treatments for major depressive disorder. The faster nonresponse in a patient is identified, the thinking goes, the faster a different therapy can be tried.
Subscribe for the latest updates from Psychiatry Advances in Motion
QEEG is the analysis of EEG through mathematical algorithms rather than visual inspection. It has been studied in psychiatry for many years and there are meaningful biological biomarkers being investigated. However, the most recent position paper on using EEG in psychiatry was published in 1991, long before modern computing power made QEEG possible.
To provide guidance to general psychiatrists and primary care physicians, Alik Widge, MD, PhD, a researcher with the Division of Neurotherapeutics, and colleagues conducted a meta-analysis of QEEG studies published between January 2000 and November 2017. In the American Journal of Psychiatry, they warn that QEEG, as studied and published to date, is not ready for routine clinical use.
"A Broad Net"
The researchers first evaluated whether there is sufficient evidence to recommend any QEEG approach for predicting treatment response in depression. They considered all QEEG studies that included adults with any type of major depressive episode, receiving any intervention and with any methodology or EEG metric. Most articles evaluated response to medication (n = 57) or response to repetitive transcranial magnetic stimulation (n = 14). In total, 53 articles covering 57 biomarkers included sufficient information for meta-analysis.
For all biomarkers considered together, the meta-analysis suggested predictive power above chance. The estimate of sensitivity was 0.72 (95% CI, 0.67–0.76) and the estimate of specificity was 0.68 (95% CI, 0.63–0.73), which the researchers call reasonable power to predict treatment response in depressive illness.
Specific Biomarkers
The researchers complemented their broad approach with a meta-regression that investigated specific biomarkers. This analysis included the proprietary Antidepressant Treatment Response index. The results showed that no biomarker had significantly greater predictive power than any other.
Study Quality
Another primary question was whether QEEG researchers have used study designs and reporting practices that make their data reproducible and reliable.
The researchers considered three aspects of high-quality studies:
- Sample sizes (small samples inflate effect sizes, which may give a false impression of efficacy)
- Statistical correction for multiple hypothesis testing
- Cross-validation (reporting the predictive performance of an EEG algorithm on a sample of patients separate from those used to develop it)
They found that study sizes were generally small, with a median N of 25. Of the 36 studies that tested multiple features, 24 did not report the use of a statistical correction. Of 71 markers that were claimed to have significant predictive ability, only six were validated.
The researchers believe that the apparent effectiveness of QEEG, detected in their broad-based meta-analysis, was probably actually an artifact of study design and selective publication. As depicted in the Figure, funnel-plot analysis suggested that the predictive power of QEEG was driven by small studies that had strong positive results.
Results of the researchers' analysis revealed a skew toward studies with larger effect sizes, indicating that few negative or weak studies are in the published literature. They also found that there was a linear relationship between sample size and effect size (Figure 1).
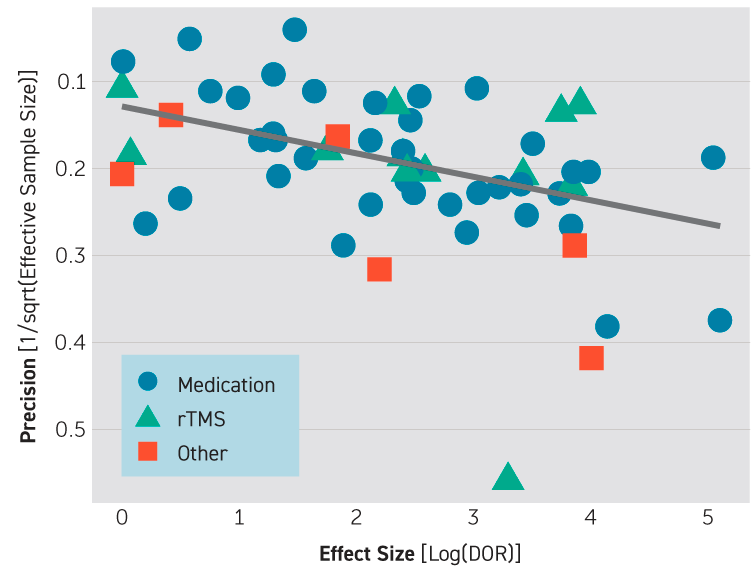
Fig. 1: Influence of Publication Bias in Results of a Meta-Analysis of Quantitative EEG (QEEG) Biomarkers in Depression Treatment
Scatterplot shows effect size of studies (log of diagnostic odds ratio) against the reciprocal of effective sample size. The overlaid line shows their linear relationship. The association of effect size with study size holds across biomarker types, reflected here by different marker shapes. rTMS=repetitive transcranial magnetic stimulation.
Conclusions
At present, marketed approaches to QEEG do not represent evidence-based care, the researchers conclude. They conclude that at present use of QEEG methods in routine clinical practice, either commercial or research-grade, is not be a wise use of health care dollars.
The authors emphasize that they are not calling for QEEG research to be stopped or slowed. Their results do not imply that QEEG findings are spurious. Rather, the problem is that links between symptom checklists and specific aspects of resting-state brain activity are not yet robust or reliable.
view original journal article Subscription may be required
Learn more about the Division of Neurotherapeutics
Learn more about depression treatment at Mass General