Can a CT tell you how to ventilate in COVID-19?
The FLARE Four
- ARDS, like much critical illness, is a heterogeneous disease. Heterogeneity is a major challenge for critical care research and clinical practice, as it is expected that patients with the same clinical syndrome may yet respond differently to the same treatment
- A large body of rigorous research exists that attempts to identify clinical, radiographic, or imaging features that predict treatment response. To date, no subphenotype has been identified that clearly predicts treatment response in a prospective trial. Interventions that have been found to reduce mortality in randomized controlled trials have been found to do so even when applied to unselected populations of patients with ARDS
- Attempts have been made to rapidly define subphenotypes in COVID-19 ARDS based on a small number of observations. An informative new report, reviewed tonight, confirms that such rapidly identified phenotypes do not accurately describe COVID-19 related ARDS and should not be used to determine treatment strategies
- Research into subphenotypes of ARDS is ongoing and will be vital to the development of specific therapies. When carefully conducted, such studies promise to overcome a central challenge in critical care research, the profound heterogeneity of our patients
Many people are still saying...COVID-19 patients have unique respiratory failure phenotypes.
Subscribe to the latest updates from FLARE Advances in Motion
Introduction
The Berlin definition of ARDS has frequently been criticized as overly inclusive (Ferguson et al., 2012). ARDS is, of course, a syndrome rather than a single disease, with attendant heterogeneity in presentation, severity and outcomes (Bellani et al., 2016). Attempts to develop diagnostic criteria have been reported to have specificity as low as ~50% when compared to a gold standard of diagnosis based on autopsy (Ferguson et al., 2005). This heterogeneity represents a significant challenge to critical care research, as a given intervention may be successful in a subgroup of patients and yet yield inconclusive or contradictory results in large trials (Iwashyna et al., 2015). As a result, researchers have long sought ways to subdivide (or “subphenotype”) ARDS patients into groups with shared physiological features that may respond to treatments similarly (Calfee et al., 2014; Prescott et al., 2016). Such efforts have generally been based on careful analysis of large datasets looking for clinical features or biomarkers that might indicate an underlying biologic or pathophysiologic similarity. Even so, to this point, there have been no prospective, randomized controlled trials showing a mortality benefit for ARDS patients selected and treated based on subphenotype. Thus personalized treatment for ARDS, prior to COVID-19, remains more of an (important) goal than an established approach.
Early in the pandemic, a division of COVID-19 ARDS into subphenotypes was proposed based entirely on qualitative descriptions of patients cared for at a single center (Gattinoni et al., 2020a, 2020b). Subsequent publications (Bhatraju et al., 2020; Grasselli et al., 2020; Ziehr et al., 2020) reporting clinical and physiological characteristics of critically ill COVID-19 patients have failed to validate these subphenotypes, but discussions persist. In part this is due to a perception that COVID-19 patients do present with imaging abnormalities that are unexpectedly mild compared to prior experience (Bernheim et al., 2020) despite evident clinical severity. It has been further asserted that these patients, with mild imaging abnormalities and postulated to have normal mechanics, will not benefit from standard management techniques such as low tidal volume ventilation and prone positioning (Marini and Gattinoni, 2020). Prior to testing in prospective trials, a proposed subphenotype must, at a minimum, accurately describe some feature of respiratory failure in association with COVID-19. Tonight, we report on an informative new paper that seeks to comprehensively determine the relationship between respiratory system mechanics and imaging findings on chest CT.
Type L and Type H
A novel proposed subphenotype specific to COVID-19 (Gattinoni et al., 2020a) ‘splits’ patients into two phenotypes: type “L” and type “H” (see table below). The type “L” phenotype is nominally characterized by a striking disconnect between degree of impairment of compliance (relatively mild, with Crs of 50 cm/H2O on average) and gas exchange (more severe, effective shunt fraction of 0.50 (Gattinoni et al., 2020b), with effective shunt broadly defined so as to include low V/Q as well as true shunt). It has further been suggested that these distinctions correspond with the extent of CT scan abnormality, and that clues from the CT scan may be used to inform ventilator management.

Table 1
Characteristics of the proposed Type L and Type H respiratory failure (Gattinoni et al., 2020a)
Though this classification system has received much attention, few data supporting its accuracy have been published to this point. The hypothesis articulated by Gattinoni and colleagues leads immediately to the question of whether the degree of abnormality on CT scan in COVID-19 had any relationship to respiratory system mechanics. This is the subject of tonight’s paper.
Do CT Scan Findings Predict Respiratory Mechanics in COVID-19 Patients?
In an insightful recent study, Bos and colleagues present a retrospective analysis of 38 critically-ill, intubated COVID-19 patients with chest CT imaging (Bos et al., 2020). Patients were ventilated using low tidal volume ventilation (6 ml/kg) and PEEP ~10 cmH2O. For each patient, the fraction of consolidated lung by CT scan was determined by classifying all areas above -500 Hounsfield units (Gattinoni et al., 2006) as consolidated, and then binning patients in quartiles of fraction of involved lung (0-25-50-75-100%). Radiographic phenotype was further classified as “focal” or “non-focal” by previously-described methods (Constantin et al., 2019). Respiratory system compliance (Crs) was calculated for each patient immediately after intubation, and a scatter plot was generated, where each point represents one patient:
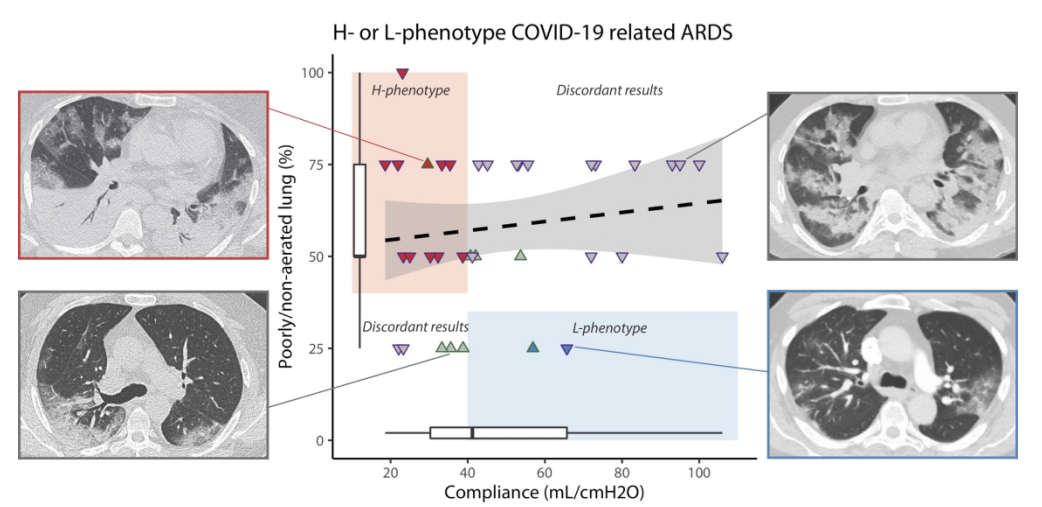
Figure 1
Comparison of respiratory system compliance versus percentage of lung that is poorly or non-aerated. Compliance of the lung is not correlated to lung aeration. Representative CT scans are shown, corresponding to H and L phenotypes. Most patients had discordant phenotypes (gray area and gray triangles). Adapted from Bos et al., 2020.
Notably, the respiratory system compliance was similar to prior reported cohorts of COVID-19 patients (Bhatraju et al., 2020; Ziehr et al., 2020), and to non-COVID-19 ARDS cohorts (Bellani et al., 2016). In this first rigorous test of the L and H subphenotypes, the authors report no relationship between respiratory system compliance and degree of aeration on CT scan. The figure above, reproduced from the paper, nicely illustrates this point. The L - phenotype should correspond to high compliance and low degree of consolidation (right lower quadrant) and the H phenotype should correspond to low compliance and high degree of consolidation (left upper quadrant) but in fact both the left lower and right upper quadrant are significantly populated. In other words, the degree of consolidation/non-aerated tissue does not predict compliance.
The authors acknowledge several limitations to their study. First, CT analysis is semi-quantitative. Second, there was no assessment of recruitability in these patients. Third, the total number of patients is relatively small.
Can We Use CT Scan Findings to Guide Ventilation Strategies in ARDS?
The failure of a particular proposed classification should not be used to criticize the entire endeavor of subphenotyping. Indeed, the suspicion that patients with more radiographically limited disease (“focal” ARDS) may respond differently to treatment is not new. It has been hypothesized that the observed lack of efficacy (and potential harm) associated with personalized “open lung” approaches in unselected patients may lie with the variable degrees of recruitability in patients with focal versus diffuse disease (Brower et al., 2004; Writing Group for the Alveolar Recruitment for Acute Respiratory Distress Syndrome Trial (ART) Investigators et al., 2017). To address whether CT imaging might identify patients with ARDS subphenotypes amenable to personalized therapy, Constantin and colleagues conducted a randomized controlled trial (Constantin et al., 2019), the LIVE trial, prior to the COVID-19 pandemic.
LIVE compared standard ventilation strategies (control) to those personalized based on imaging findings (focal versus non-focal) (figure 2). The control group was treated with standard lung protective ventilation (tidal volume 6 ml/kg, PEEP set according to the low PEEP, high FiO2 ARDSnet table (Brower et al., 2004; Writing Group for the Alveolar Recruitment for Acute Respiratory Distress Syndrome Trial (ART) Investigators et al., 2017) and encouragement of prone positioning). Patients in the personalized group were subdivided according to CT scan morphology into focal (opacities localized to dependent and posterior portions of the lung) and non-focal groups. Focal patients received a larger tidal volume (8cc/kg IBW), mandatory prone positioning and recruitment maneuvers only as a rescue. The non-focal group was treated with tidal volume 6 ml/kg, PEEP to reach a plateau pressure of 30 cmH2O and mandatory recruitment maneuvers, with prone positioning as a rescue strategy. In contrast to the L and H schema, this protocol effectively postulates that recruitability is orthogonal to degree of consolidation on CT. In other words - patients with focal disease may be best recruited by prone ventilation while those with non-focal disease may benefit more from formal recruitment maneuvers. In this way, different ventilation and recruitment strategies were prescribed for different imaging findings.
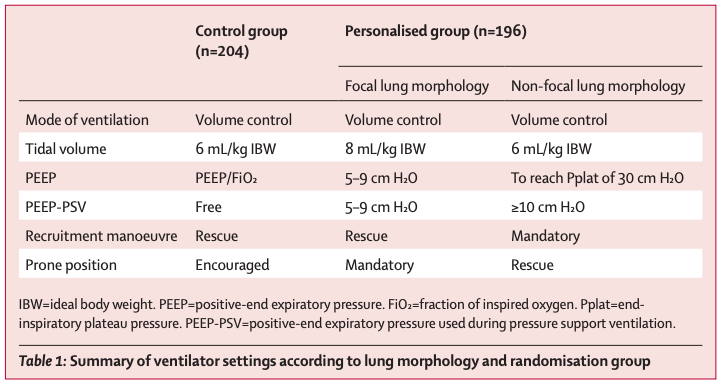
Figure 2
Summary of ventilator settings and strategies used in the LIVE trial (Constantin et al., 2019).
Overall, there was no difference in mortality between the groups in an intention to treat analysis. However, the interpretation of this result is complicated by the fact that in a post hoc analysis of imaging, it was found that a significant percentage of patients were misclassified into focal or diffuse. Interestingly, there was a statistically significant interaction between misclassification and mortality, and when mortality was analyzed per protocol there was a mortality benefit to personalized therapy compared to the control group. These findings emphasize the importance of appropriate subphenotyping, and, paradoxically, the difficulty of doing so accurately. LIVE suggests that there may in fact be a useful subphenotyping of ARDS based on imaging findings. Notably, the ventilation approaches in LIVE do not correlate with the proposed strategies for L and H subphenotypes; for example all patients in LIVE were considered potentially recruitable with either prone positioning or a recruitment maneuver. In addition, as LIVE was conducted prior to the emergence of COVID-19, the existence of imaging-based phenotypes (even if confirmed by careful analysis in a way that the L and H phenotypes have not been) are not likely to be informative as to the specific to the biology of COVID-19.
Conclusions
In a timely and informative manuscript, Bos and colleagues describe heterogeneity in lung morphology in COVID-19-associated ARDS. Their findings are consistent with prior reports on chest CT imaging in COVID-19 (reviewed in the March 26 FLARE). The authors additionally validate the heterogeneity of respiratory system compliance in COVID-19 ARDS patients, also consistent with prior reports (and covered most recently in the May 13 FLARE). After matching patient respiratory system compliance with radiographic findings, the authors were not able to subphenotype patients consistently into the previously-proposed COVID-19 ARDS “H” and “L” subphenotypes. Based on this study and prior studies, we discourage clinicians from assuming respiratory system mechanics correlate with CT scan patterns, and advocate for bedside measurement and titration of ventilator settings based on individually measured mechanics.
References:
- Bellani, G., Laffey, J.G., Pham, T., Fan, E., Brochard, L., Esteban, A., Gattinoni, L., van Haren, F., Larsson, A., McAuley, D.F., et al. (2016). Epidemiology, Patterns of Care, and Mortality for Patients With Acute Respiratory Distress Syndrome in Intensive Care Units in 50 Countries. JAMA 315, 788–800.
- Bernheim, A., Mei, X., Huang, M., Yang, Y., Fayad, Z.A., Zhang, N., Diao, K., Lin, B., Zhu, X., Li, K., et al. (2020). Chest CT Findings in Coronavirus Disease-19 (COVID-19): Relationship to Duration of Infection. Radiology 200463.
- Bhatraju, P.K., Ghassemieh, B.J., Nichols, M., Kim, R., Jerome, K.R., Nalla, A.K., Greninger, A.L., Pipavath, S., Wurfel, M.M., Evans, L., et al. (2020). Covid-19 in Critically Ill Patients in the Seattle Region - Case Series. N. Engl. J. Med.
- Bos, L.D.J., Paulus, F., Vlaar, A.P.J., Beenen, L.F.M., and Schultz, M.J. (2020). Subphenotyping ARDS in COVID-19 Patients: Consequences for Ventilator Management. Annals of the American Thoracic Society.
- Brower, R.G., Lanken, P.N., MacIntyre, N., Matthay, M.A., Morris, A., Ancukiewicz, M., Schoenfeld, D., Thompson, B.T., and National Heart, Lung, and Blood Institute ARDS Clinical Trials Network (2004). Higher versus lower positive end-expiratory pressures in patients with the acute respiratory distress syndrome. N. Engl. J. Med. 351, 327–336.
- Calfee, C.S., Delucchi, K., Parsons, P.E., Thompson, B.T., Ware, L.B., Matthay, M.A., and NHLBI ARDS Network (2014). Subphenotypes in acute respiratory distress syndrome: latent class analysis of data from two randomised controlled trials. Lancet Respir Med 2, 611–620.
- Constantin, J.-M., Jabaudon, M., Lefrant, J.-Y., Jaber, S., Quenot, J.-P., Langeron, O., Ferrandière, M., Grelon, F., Seguin, P., Ichai, C., et al. (2019). Personalised mechanical ventilation tailored to lung morphology versus low positive end-expiratory pressure for patients with acute respiratory distress syndrome in France (the LIVE study): a multicentre, single-blind, randomised controlled trial. Lancet Respir Med 7, 870–880.
- Ferguson, N.D., Frutos-Vivar, F., Esteban, A., Fernández-Segoviano, P., Aramburu, J.A., Nájera, L., and Stewart, T.E. (2005). Acute respiratory distress syndrome: underrecognition by clinicians and diagnostic accuracy of three clinical definitions. Crit. Care Med. 33, 2228–2234.
- Ferguson, N.D., Fan, E., Camporota, L., Antonelli, M., Anzueto, A., Beale, R., Brochard, L., Brower, R., Esteban, A., Gattinoni, L., et al. (2012). The Berlin definition of ARDS: an expanded rationale, justification, and supplementary material. Intensive Care Med. 38, 1573–1582.
- Gattinoni, L., Caironi, P., Cressoni, M., Chiumello, D., Ranieri, V.M., Quintel, M., Russo, S., Patroniti, N., Cornejo, R., and Bugedo, G. (2006). Lung recruitment in patients with the acute respiratory distress syndrome. N. Engl. J. Med. 354, 1775–1786.
- Gattinoni, L., Chiumello, D., Caironi, P., Busana, M., Romitti, F., Brazzi, L., and Camporota, L. (2020a). COVID-19 pneumonia: different respiratory treatments for different phenotypes? Intensive Care Med.
- Gattinoni, L., Coppola, S., Cressoni, M., Busana, M., Rossi, S., and Chiumello, D. (2020b). Covid-19 Does Not Lead to a “Typical” Acute Respiratory Distress Syndrome. Am. J. Respir. Crit. Care Med.
- Grasselli, G., Zangrillo, A., Zanella, A., Antonelli, M., Cabrini, L., Castelli, A., Cereda, D., Coluccello, A., Foti, G., Fumagalli, R., et al. (2020). Baseline Characteristics and Outcomes of 1591 Patients Infected With SARS-CoV-2 Admitted to ICUs of the Lombardy Region, Italy. JAMA.
- Iwashyna, T.J., Burke, J.F., Sussman, J.B., Prescott, H.C., Hayward, R.A., and Angus, D.C. (2015). Implications of Heterogeneity of Treatment Effect for Reporting and Analysis of Randomized Trials in Critical Care. Am. J. Respir. Crit. Care Med. 192, 1045–1051.
- Marini, J.J., and Gattinoni, L. (2020). Management of COVID-19 Respiratory Distress. JAMA.
- Prescott, H.C., Calfee, C.S., Thompson, B.T., Angus, D.C., and Liu, V.X. (2016). Toward Smarter Lumping and Smarter Splitting: Rethinking Strategies for Sepsis and Acute Respiratory Distress Syndrome Clinical Trial Design. Am. J. Respir. Crit. Care Med. 194, 147–155.
- Writing Group for the Alveolar Recruitment for Acute Respiratory Distress Syndrome Trial (ART) Investigators, Cavalcanti, A.B., Suzumura, É.A., Laranjeira, L.N., Paisani, D. de M., Damiani, L.P., Guimarães, H.P., Romano, E.R., Regenga, M. de M., Taniguchi, L.N.T., et al. (2017). Effect of Lung Recruitment and Titrated Positive End-Expiratory Pressure (PEEP) vs Low PEEP on Mortality in Patients With Acute Respiratory Distress Syndrome: A Randomized Clinical Trial. JAMA 318, 1335–1345.
- Ziehr, D.R., Alladina, J., Petri, C.R., Maley, J.H., Moskowitz, A., Medoff, B.D., Hibbert, K.A., Thompson, B.T., and Hardin, C.C. (2020). Respiratory Pathophysiology of Mechanically Ventilated Patients with COVID-19: A Cohort Study. Am. J. Respir. Crit. Care Med.
Learn more about research in the Division of Pulmonary and Critical Care Medicine
View all COVID-19 updates