Artificial Intelligence Estimates Biological Age, Predicts Mortality From a Chest X-ray
Key findings
- This study introduces a convolutional neural network, dubbed CXR-Age, that estimates biological age from a chest X-ray
- CXR-Age was developed in 116,035 individuals and validated in independent testing datasets from the Prostate, Lung, Colorectal, Ovarian (PLCO) Cancer Screening Trial (n=40,967) and National Lung Screening Trial (n=5,414)
- A five-year increase in CXR-Age carried a higher risk of all-cause mortality and cardiovascular mortality than a five-year increase in chronological age
- CXR-Age was additive to traditional cardiovascular risk factors and radiographic findings
- Additional work now underway is exploring whether substituting CXR-Age for chronological age can improve age-based cardiovascular risk scores and age-based recommendations for lung cancer screening
Because people age at various rates, various measures of biological age have been proposed as alternatives to chronological age for understanding overall health and predicting longevity. A familiar example is vascular age, which is assessed with imaging markers such as coronary artery calcium score or carotid intimal medial thickness.
Subscribe to the latest updates from Radiology Advances in Motion
Researchers at the Massachusetts General Hospital have introduced a new measure of biological age, dubbed CXR-Age, which is estimated by having a convolutional neural network (CNN) analyze a chest X-ray image. CNNs, a form of artificial intelligence, "learn" to extract patterns from medical imaging data.
In JACC: Cardiovascular Imaging, Vineet K. Raghu, PhD, instructor in Radiology at Mass General, Jakob Weiss, MD, senior resident in Radiology, Michael T. Lu, MD, PhD, co-director of the Cardiovascular Imaging Research Center, and colleagues show CXR-Age is better than chronological age at predicting long-term all-cause and cardiovascular mortality.
Methods
CXR-Age was developed in two stages using chest X-rays from 116,035 individuals:
- Stage 1—A CNN was trained to estimate chronological age from radiographs
- Stage 2—The stage 1 model was used to train CXR-Age to estimate biological age from chest X-rays taken on 25% of the intervention arm in the Prostate, Lung, Colorectal, Ovarian (PLCO) Cancer Screening Trial
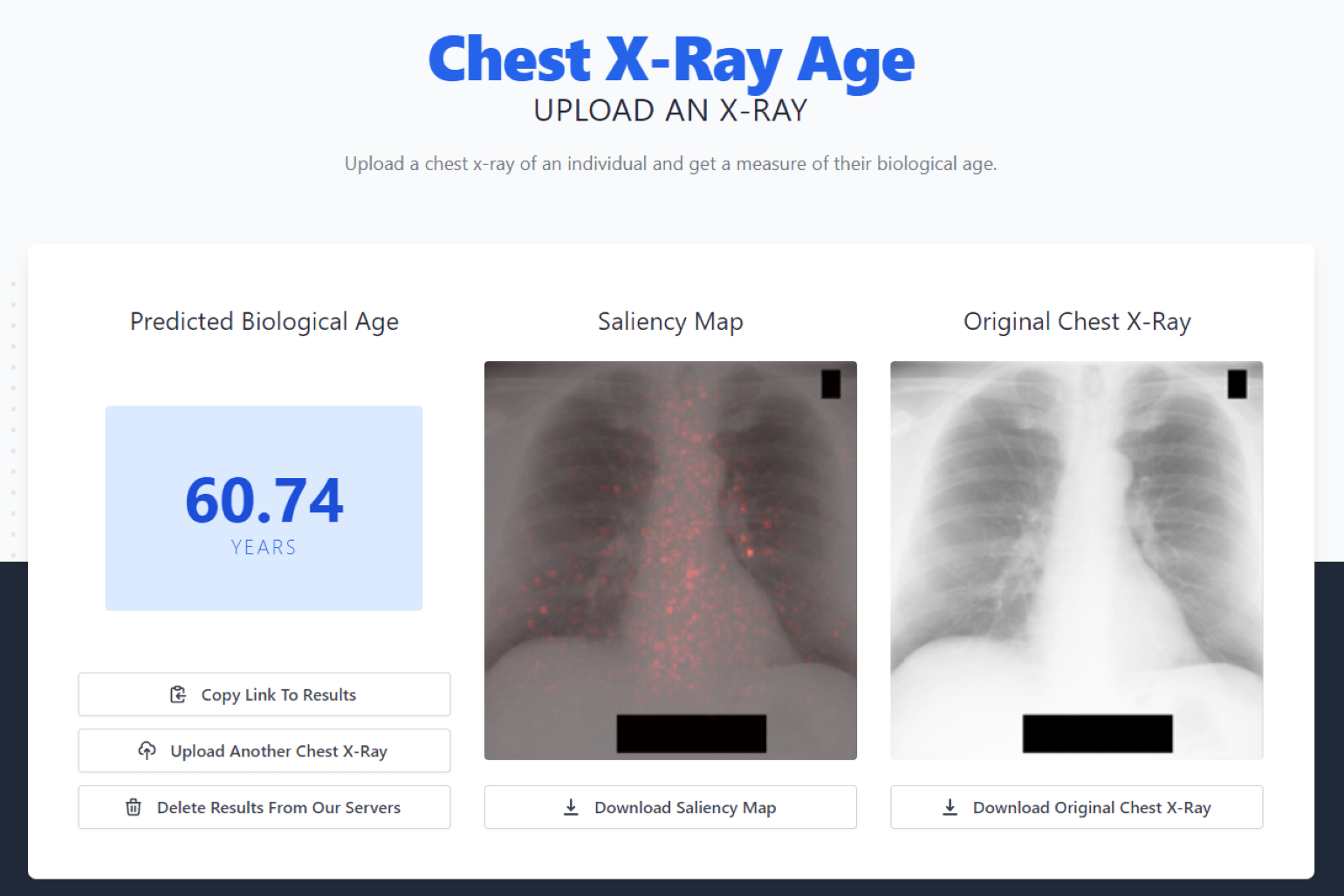
Figure 1
The free cxrage.org website calculates CXR-Age from uploaded chest x-ray images.
CXR-Age was validated in two datasets not used for model development:
- The remaining 75% of participants in the PLCO intervention arm (n=40,967; mean age, 63; median follow-up, 17 years)
- Baseline chest X-rays from the National Lung Screening Trial (NLST, n=5,414; mean age, 62; median follow-up, 12 years)
All-Cause Mortality
A five-year increase in CXR-Age carried a higher risk of all-cause mortality than a five-year increase in chronological age did:
- PLCO—HR for mortality, 2.26 for CXR-Age vs. 1.77 for chronological age (P<0.001)
- NLST—1.82 vs. 1.49 (P<0.001)
The results were additive to baseline clinical risk factors and radiographic findings.
Cardiovascular Mortality
Similarly, a five-year increase in CXR-Age carried a higher risk of cardiovascular mortality (observed over 13 years of follow-up) compared with a five-year increase in chronological age:
- PLCO—HR for mortality, 2.45 for CXR-Age vs. 1.82 for chronological age
- NLST—1.94 vs. 1.59
These results were additive to clinical risk factors.
Looking Ahead
A major advantage of framing risk as a biological age (e.g., biological age of 68 at a chronological age of 55) is that patients can more easily grasp differences in age than risk score probabilities. Since chest radiography is one of the most common diagnostic tests there would be ample opportunities to use CXR-Age.
The researchers are now exploring whether substituting CXR-Age for chronological age can improve age-based cardiovascular risk scores and age-based recommendations for lung cancer screening. To encourage other investigations, they have published the CXR-Age model as free open-source software. They have also set up a free website where users can run CXR-Age on uploaded chest X-rays.
view original journal article Subscription may be required
Learn more about the Cardiovascular Imaging Research Center
Learn more about the Department of Radiology